The 1st Video Scene Parsing in the Wild Challenge Workshop
16 October, 2021
ICCV 2021, VIRTUAL
Schedule [Live Zoom Link ]
12:00 PM (EDT Time) Chairs’ opening remarks
12:15 PM (EDT Time) Liang-Chieh (Jay) Chen, Google AI [youtube] [bilibili]
12:45 PM ‪(EDT Time) Hengshuang Zhao, University of Oxford [youtube ]
1:15 PM (EDT Time) Federico Perazzi, Bending Spoons [youtube ] [bilibili ]
1:45 PM (EDT Time) Challenge 1st place Winners’ Oral Presentation [youtube ][bilibili ]
2:00 PM (EDT Time) Challenge 3rd place Winners’ Oral Presentation [youtube ][bilibili ]
2:15 PM (EDT Time) Concluding remarks
Introduction
Scene parsing is one of the main problems in computer vision, which aims at recognizing the semantics of each pixel in the given image. Recently, several image-based datasets have been collected to evaluate the effectiveness of scene parsing approaches. However, since the real-world is actually video-based rather than a static state, learning to perform video scene parsing is more reasonable and practical for realistic applications. Although remarkable progress has been made in image-based scene parsing, few works have been proposed to consider the video scene parsing, which is mainly limited by the lack of suitable benchmarks. To advance the scene parsing task from images to videos, we present a new dataset (VSPW dataset) and a competition in this workshop, aiming at performing the challenging yet practical Video Scene Parsing in the Wild (VSPW).
Video Scene Parsing aims to assign pre-defined semantic labels to pixels of all frames in a given video, which brings new challenges compared with image semantic segmentation. One main challenge of Video Scene Parsing task is how to leverage the temporal information for high predictive accuracy. We expect the challengers to provide results in terms of the accuracy better than image-based semantic segmentation methods.
Invited Speakers
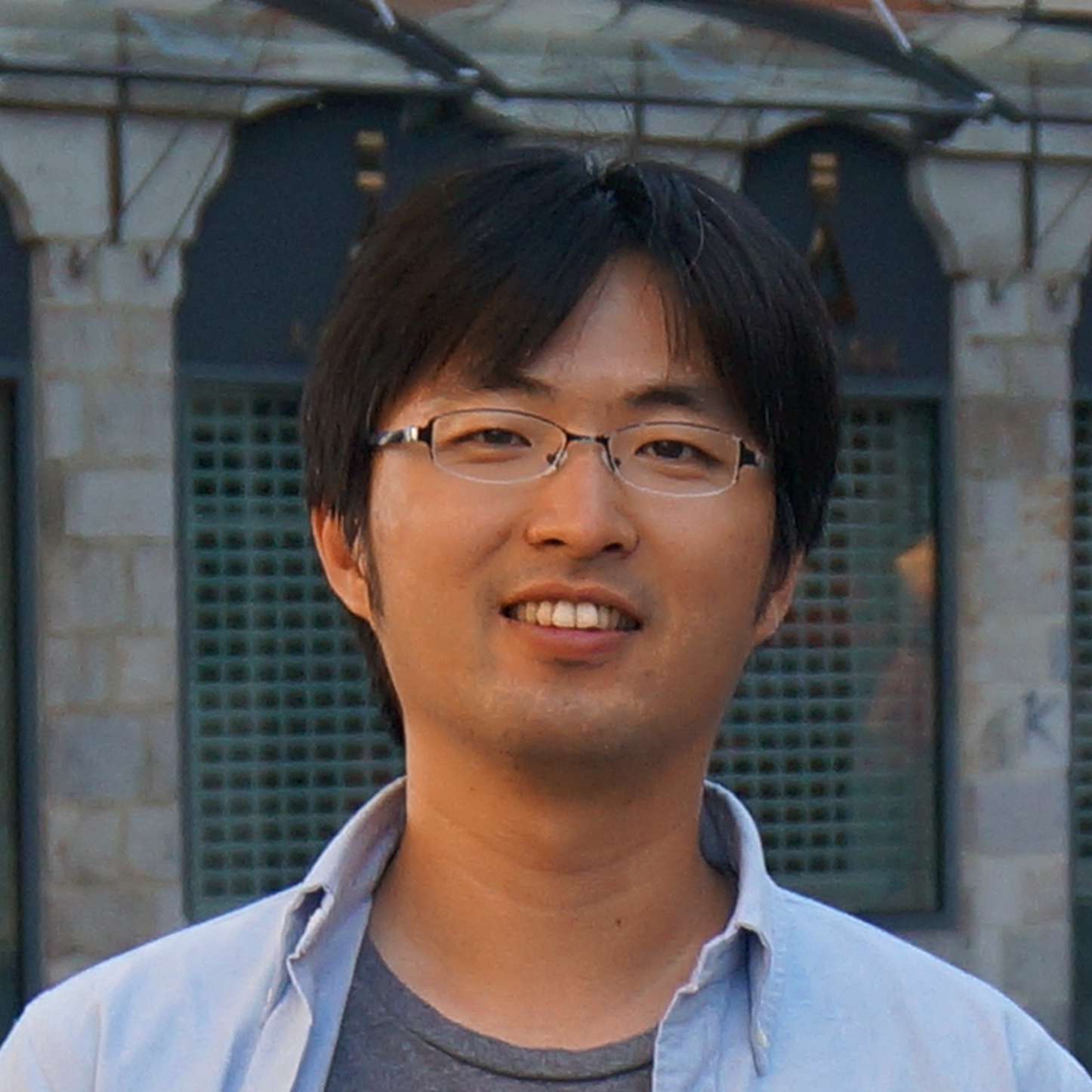
Liang-Chieh (Jay) Chen
Research Scientist at Google AI
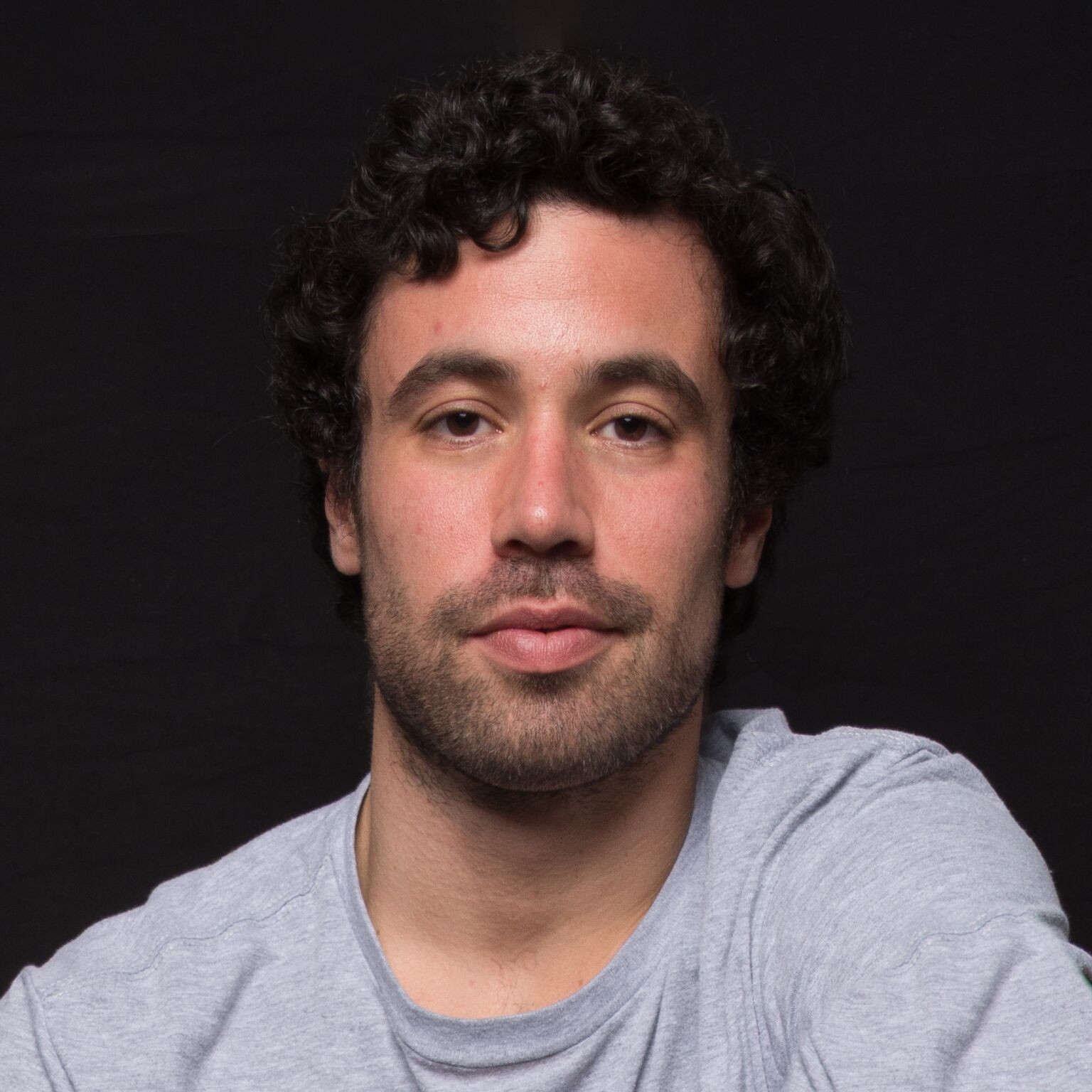
Federico Perazzi
Research Scientist and Head of AI at Bending Spoons
Organizers
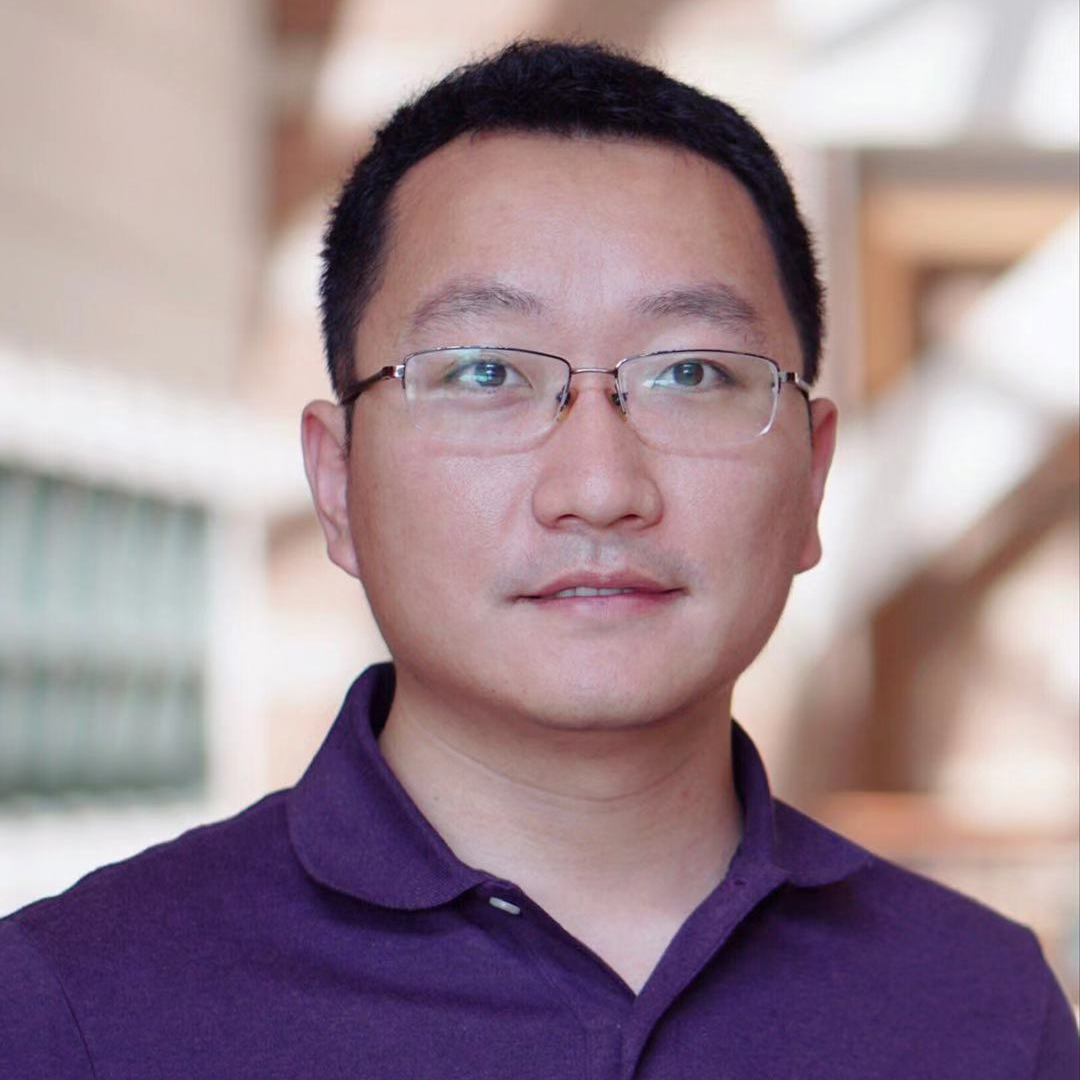
Yunchao Wei
University of Technology Sydney
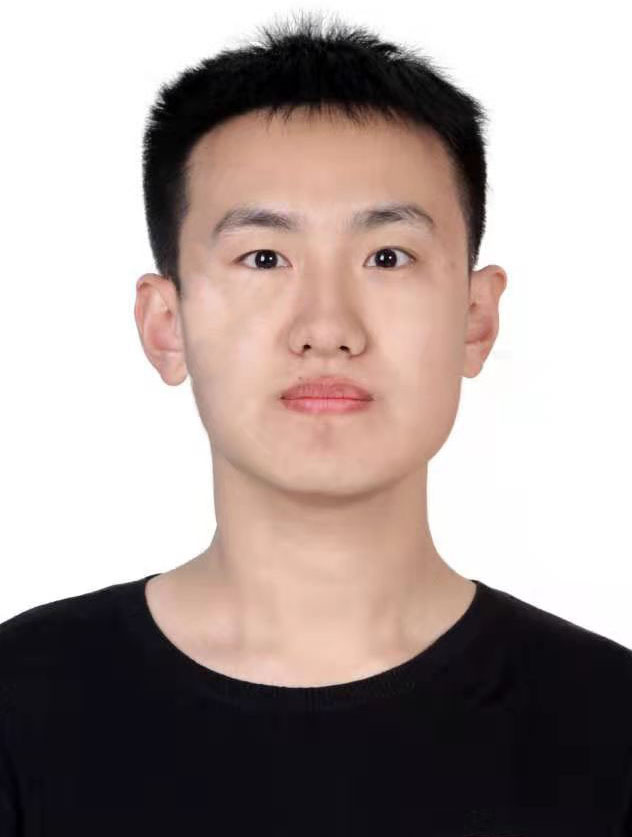
Jiaxu Miao
Zhejiang University
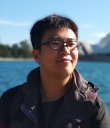
Yu Wu
University of Technology Sydney
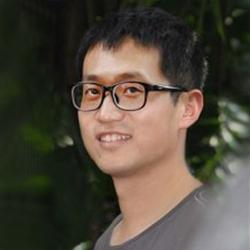
Yi Yang
Zhejiang University
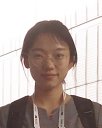
Si Liu
Beihang University
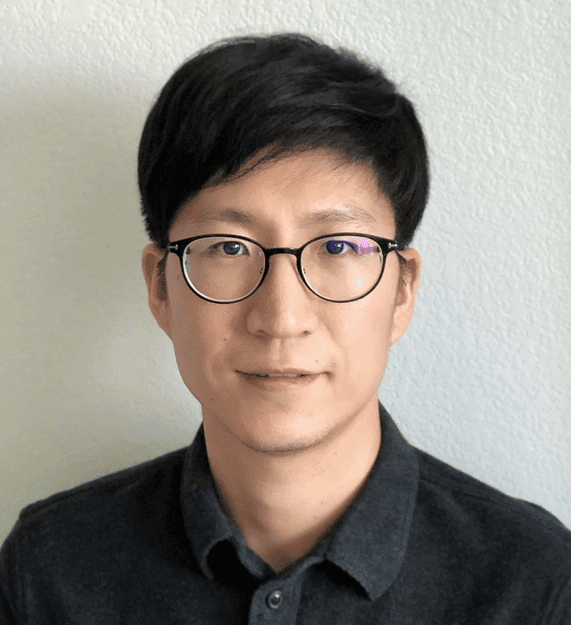
Zhu Yi
Amazon
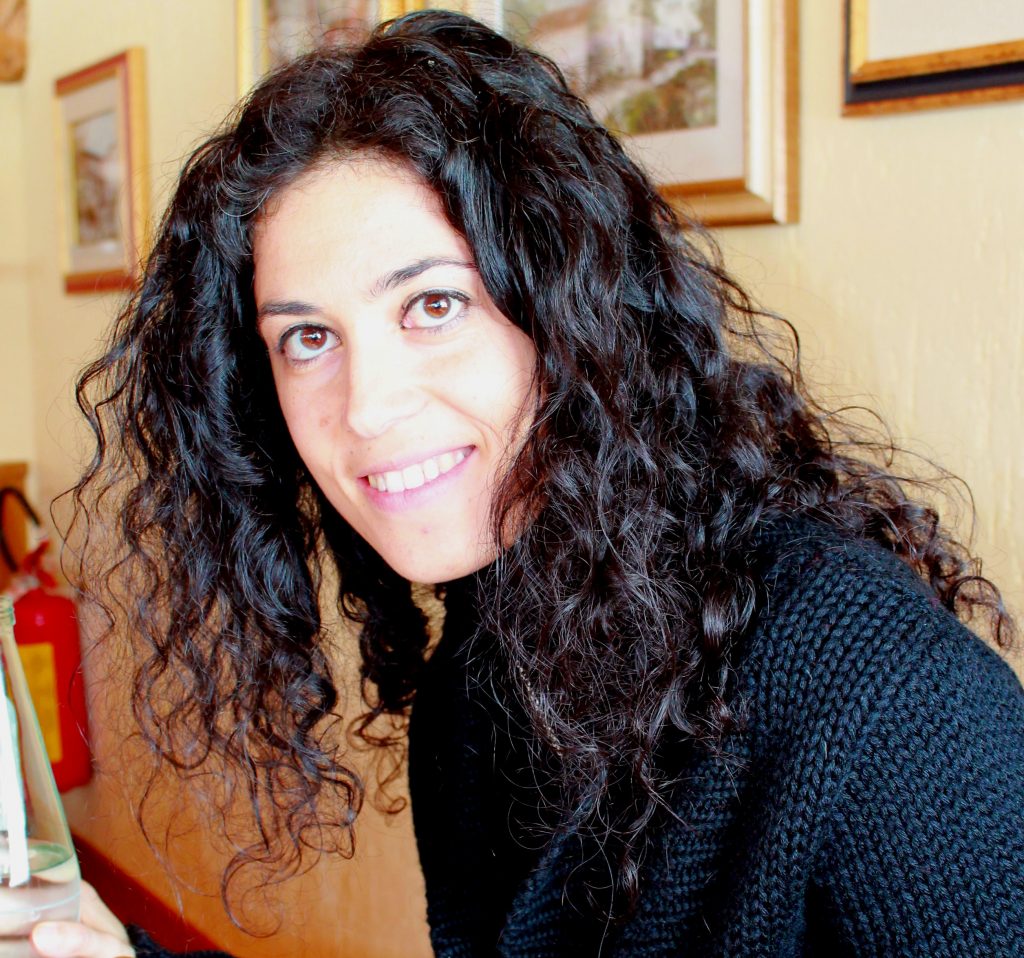
Elisa Ricci
University of Trento
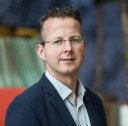
Cees Snoek
University of Amsterdam